Good forecasting thrives on a delicate balance of math, expertise, and…vibes.
Predicting the future is difficult, but not impossible — and some people are much better at it than others. This insight has spawned a community dedicated to developing better and better methods of forecasting. But while our techniques have become increasingly sophisticated, even the best forecasters still make mistakes.
In my work as an analyst for the Forecasting Research Institute, and as a member of the forecasting collective Samotsvety, I’ve had plenty of opportunities to see how forecasters err. By and large, these mistakes fall into two categories. The first mistake is in trusting our preconceptions too much. The more we know — and the more confident we are in our knowledge — the easier it is to dismiss information that doesn’t conform to the opinions we already have. But there’s a more insidious second kind of error that bites forecasters — putting too much store in clever models that minimize the role of judgment. Just because there’s math doesn’t make it right.
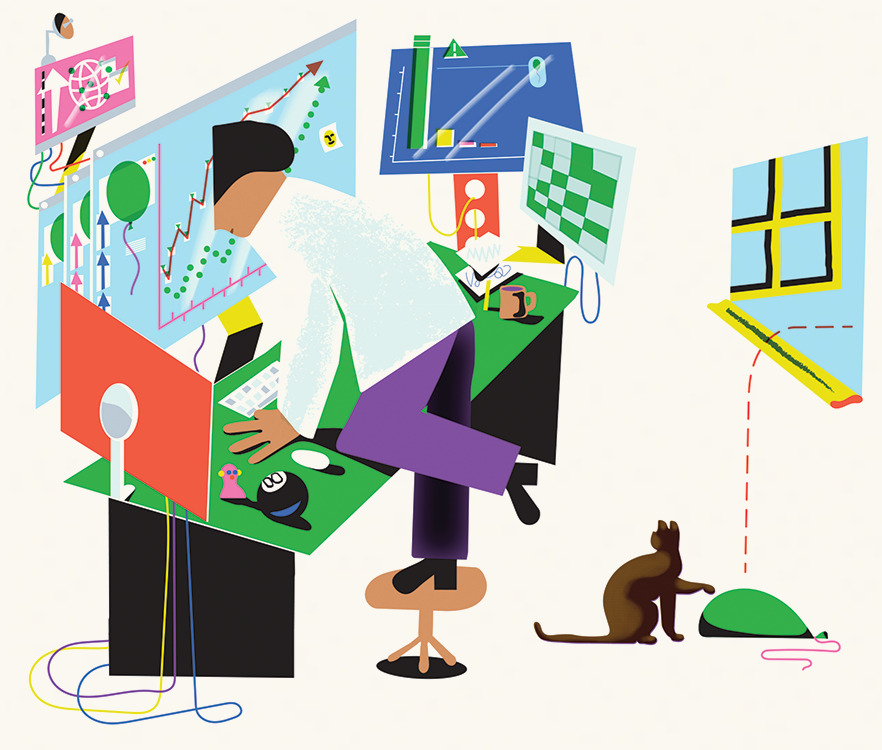
Forecasters versus experts
The first scientific study of judgmental 1 forecasting was conducted in the 1960s by a gentleman at the CIA named Sherman Kent. Kent noticed that in their reports, intelligence analysts used imprecise phrases like “we believe,” “highly likely,” or “little chance.” He wanted to know how the people reading the reports actually interpreted these phrases. He asked 23 NATO analysts to convert the phrases into numerical probabilities, and their answers were all over the place — “probable” might mean a 30% chance to one person and an 80% chance to another. Kent advocated the use of few consistent odds expressions in intelligence reports, but his advice was largely ignored. It would take another two decades for the intelligence community to seriously invest in the study of prediction.
The modern forecasting community largely emerged from the work of one man: Philip Tetlock. In 1984, Tetlock, then a professor of political science at UC Berkeley, held his first forecasting tournament. His goal was to investigate whether experts — government officials, journalists, and academics — were better at making predictions in their areas of interest than intelligent laypeople. Over the next two decades, Tetlock asked both experts and informed laypeople to make numerical predictions of the likelihoods of specific events. The results were published in his 2005 book, Expert Political Judgment: How Good Is It? How Can We Know? The upshot: Experts make for terrible forecasters. 2
Tetlock’s work helped inspire the ACE Program forecasting tournament run by the Intelligence Advanced Research Projects Activity (IARPA), a research arm of the American intelligence community. In the first two years of the tournament, Tetlock’s team won so handily that IARPA canceled the remaining competitions.
If expertise doesn’t make for accurate predictions, then what does? Among other things, the best forecasters have a quality that Tetlock borrowed from the research of psychologist Jonathan Baron: “active open-mindedness.” Instead of operating on autopilot, those who score high in active open-mindedness take into consideration evidence that goes against their beliefs, pay attention to those who disagree with them, and are willing — even eager — to change their minds. It can be particularly difficult for subject matter experts, who may be heavily invested in particular narratives, to fit new evidence into their existing worldview. It’s active open-mindedness that separates these “superforecasters” from the chaff.
What about superforecasters who are also subject matter experts? Do their forecasting skills help them correct for their ingrained biases? Anecdotal evidence suggests not. Marc Koehler is senior vice president of Good Judgment Inc. He started forecasting as part of IARPA’s ACE Program after having served as a U.S. diplomat in Asia and working on China issues for many years. He probably knew more about China and U.S.-China relations than anyone else on the platform. He’s exactly the person you want on your team when China questions come up at trivia night. Naïvely, you might think — as Marc thought — that he’d be very good at forecasting on China-related questions.
As Marc will tell you, he bombed on China questions. In fact, he did much better in forecasting events related to Africa than to China, despite never having worked on African issues. He was actually quite good on non-China questions — so good that he placed in the top 2% of participants in the ACE Program, earning the title of Superforecaster. (Humbled, he did also improve on China questions over time.)
According to Marc, it took “some serious de-biasing work” before his performance on China questions caught up with his performance on topics he knew less about. Many superforecasters who also have expertise in a particular area say the same. Detailed knowledge of a particular topic makes it harder to recognize when new events undermine their detailed models of the world. Expertise isn’t useless. It’s essential for understanding and contextualizing events. But the further in the future one tries to look, the more that confidence in one’s own knowledge can mislead.
Some forecasters take this lesson to extremes: Every time they read a headline related to something they’ve predicted, they’ll reevaluate their whole forecast. This is justified, at times. If some shocking event upends your view of the world, maybe you should tear down your model and start from scratch. But it’s also easy to overcorrect.
One example of collective overcorrection happened on INFER, a forecasting platform used by the U.S. government. The question: Will Myanmar hold national elections on or before 31 December 2023? Around February of this year, forecasters on the platform noticed that Myanmar’s ruling junta had posted new rules for elections and announced plans to start testing new voting machines. Some forecasters made larger updates, for example by doubling their probability that an election would occur (e.g., 22% to 45%). That accounts for the climb of the crowd aggregate from 35% on February 10 to 58% on March 6 — the highest it would be for the rest of the year. Without any further signs of elections, the forecast gradually readjusted. As of this writing on December 11, current forecasts are at roughly 0%.
My friend Chinmay Ingalagavi, a fellow member of Samotsvety, provided this Myanmar example. He described the temptation to overcorrect as crowd forecasts change. “When a new piece of info comes out and everyone around is updating their forecasts by large amounts, it’s easy to forget how much I’d already incorporated this possibility into my model while making the last forecast,” he said. “I get caught up with everyone else.”
In summary, to be a great forecaster, you should know something about the thing you’re forecasting — but not too much, lest it bias your judgment. It’s important to keep an open mind, but just as important to avoid wild swings over news that may be less surprising than it initially seems. What’s a would-be forecaster to do? The answer — many would say — is to shut up and multiply.
Shut up and multiply
Experienced forecasters like to use quantitative models to make their predictions. 3 For complicated questions, this usually involves picking a decomposition — that is, a way of breaking down a hard problem into smaller, more manageable pieces. For example, a forecaster interested in the results of the next presidential election might want to think about the odds of a particular candidate winning their party’s primary, the likelihood of a major scandal, or the probability that unemployment might rise or inflation might fall. Decomposing questions like this is a major part of forecasting, and when it’s done well, it can help ground our intuitions and keep our biases in check. Good arguments about forecasts often revolve around decompositions — we may agree on the component forecasts but disagree on how they’re strung together.
As an example of how a bad decomposition can skew a forecast, let’s take another INFER question: Will Xi Jinping be general secretary of the Chinese Communist Party’s Central Committee on December 31, 2022? One way to approach this is to think of all the ways Xi Jinping could have ceased to be general secretary of the CCP by the end of the year. Try it, it’s fun. You can think of loads of ways! 1) He could die. 2) He could decide he wants a different title (there was some talk of his bringing back the mantle of “chairman”). 3) He could get deposed. 4) He could wake up one day and say, “I’ve had enough. Time to retire.” The list goes on and on. None of these are very likely, but even sub-1% probabilities add up.
If any one of these things happened, Xi Jinping would stop being general secretary, and they’re not all mutually exclusive. This makes our decomposition very simple: We take all our small probabilities and calculate the odds that none of them happen.
That’s exactly what I did — and in September 2022, when the crowd aggregate was at 94%, I forecasted 72% that Xi Jinping would still be general secretary on December 31. While it’s true that you can’t judge a person based on one forecast, I think we can all agree this was a bad call on my part. Trying to come up with ways Xi might cease to be chairman wasn’t a bad idea — it’s often a useful tool — but I failed to consider how easy it was to come up with arbitrarily many of them and how difficult it is to guess the true likelihood of highly unlikely events. 4 Probabilities add up, yes — but they might add up to a very small number.
A very different sort of decomposition that’s gotten attention recently comes from Joe Carlsmith’s report Is Power-Seeking AI an Existential Risk?, which asks whether AI will cause an existential catastrophe by 2070. To put it much too simply (I encourage everyone to read the original report), for AI to pose an existential threat, it would require six ingredients, in this order:
1) Feasibility: It will be feasible to create powerful enough AI systems.
2) Inventives: People will want to build them.
3) Alignment difficulty: It’ll be harder to build good ones than bad ones.
4) High-impact failure: Some of those bad ones will try to get power over people.
5) Disempowerment: And at least some of those will succeed at seizing power from humans…
6) … To the extent that it will constitute an existential catastrophe.
Carlsmith assigns a probability to each of these conditions and multiples them together to arrive at his final result — a 5% chance of existential catastrophe.
But “existential catastrophe” is a speculative thing, and there are lots of possible ways to break down the question. When Samotsvety did AI risk forecasts last year, we went through the exercise of using several decompositions. My answers were very different depending on the decomposition — in fact, they weren’t even in the same ballpark. Using Carlsmith’s model, I arrived at a 16.1% chance of existential catastrophe (I assigned different probabilities to some of the components than he did). But using my colleague Jonathan Mann’s (also plausible!) decomposition got me a 1.7% chance.
My forecasts using Carlsmith’s decomposition
Feasibility: 80.0%
Incentives | Feasibility: 95.0%
Alignment Difficulty | (Feasibility, Incentives): 80.0%
High-Impact Failures | (Alignment Difficulty, Feasibility, Incentives): 60.0%
Disempowerment | (High-Impact Failures, Alignment Difficulty, Feasibility, Incentives): 55.0%
Catastrophe | All of the above: 80.0%
Existential Risk: 16.1%
My forecasts using Jonathan Mann (Samotsvety)’s decomposition
Motive: AI will have the motive to cause doom: 30.4%
Means: AI will have the capability to cause doom: 10.0%
Opportunity: AI will have the opportunity to cause doom: 60.0%
Simultaneity (all three conditions on one team): 95.0%
Existential Risk: 1.7%
Of course, there are problems with both of these decompositions (perhaps you can think of a better one). One issue, raised by the forecaster Eli Lifland, is that the Carlsmith model has us thinking about the problem conjunctively: That is, for a catastrophe to happen, each condition needs to be satisfied in turn. There’s only one path toward disaster, and if any step is blocked, we’ll be safe. But we could just as easily create a model where there are many ways things could go wrong, and only a few ways where it goes right (only a few “win conditions,” as Eli put it). That model might produce a much higher probability of existential catastrophe. 5
Different decompositions can yield radically different results — so try a few and don’t be precious about your model. Average them together, give more weight to the ones that make more sense to you (in the hard sciences they call this an “ensemble model”) … and then go with your gut.
All decompositions are wrong, but some are useful, as the saying goes. Ultimately — and it pains me to say this, being a quant person myself — we can put too much store in quantitative models. Misha Yagudin, (yet another) Samotsvety forecaster and the founder of Arb Research, says getting good at forecasting is about “feeling epistemic feelings with more nuance,” and he’s absolutely right. You might find yourself thinking, “This model is so fancy, it must be right … but 10% just feels too high!” Listen to that feeling.
It’s almost always worth the effort to make a quantitative model — not because its results are the immutable truth but because practicing decomposing questions and generating specific probabilities are how you train yourself to become a better forecaster. As we’ve seen, our intuitions can lead us astray, but they’re also a valuable tool. And the more time you spend forecasting, the more reliable those intuitions will become.
I want to thank Misha Yagudin, Eli Lifland, Jonathan Mann, and Chinmay Ingalagavi for their contributions, and Astrid Sorflaten and Stewart Hickman for their helpful comments.
- In contrast to statistical forecasting, judgmental forecasting involves making predictions about future events where there is limited or no historical data available, or when the situation is so unique that past data might not be relevant. ↩
- An important caveat: Tetlock’s initial study found that experts had an overall poor prediction track record but did not directly compare them with what would become non-expert superforecasters. Other studies have found that aggregated predictions by superforecasters outperform aggregated intelligence community experts, but there is debate over whether this is due to particulars of the aggregation method used. See Arb Research’s Comparing Top Forecasters and Domain Experts and Goldstein et al’s Assessing the Accuracy of Geopolitical Forecasts from the US Intelligence Community’s Prediction Market. ↩
- You can see some of these in previous issues of Asterisk — for example, Jonathan Mann’s forecast on the impact of AI on tech jobs, Juan Cambeiro’s look at future pandemics and Jared Leibowich’s model on the progress of monkeypox. ↩
- I also made the simplifying assumption that all the possibilities I thought of were independent of one another; that is, one thing happening doesn’t influence the possibility of another thing happening. This clearly isn’t true in this case. For one thing, Xi can’t both retire and be deposed. ↩
- Carlsmith has since revised his own estimates, as he explained in a blog post earlier this year: “A few years back, I wrote a report about AI risk, where I put the probability of doom by 2070 at 5%. Fairly quickly after releasing the report, though, I realized that this number was too low. Specifically, I also had put 65% on relevantly advanced and agentic AI systems being developed by 2070. So my 5% was implying that, conditional on such systems being developed, I was going to look them in the eye and say (in expectation): ‘~92% that we’re gonna be OK, x-risk-wise.’ But on reflection, that wasn’t, actually, how I expected to feel, staring down the barrel of a machine that outstrips human intelligence in science, strategy, persuasion, power.” ↩